Jonathan Peck (he/him)
Post-doctoral researcher @ Ghent University
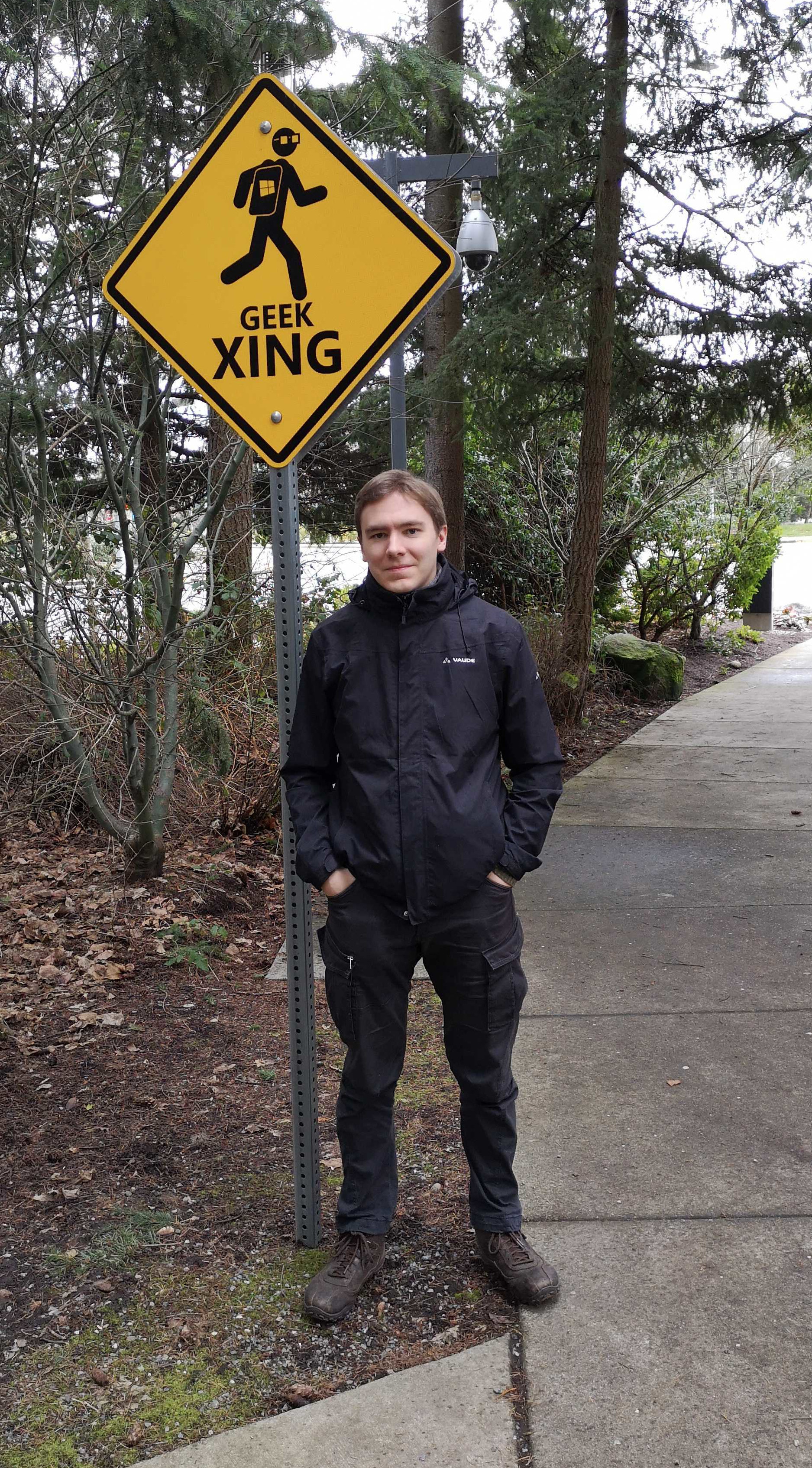
Campus Sterre
Krijgslaan 281
Gent, 9000 Belgium
I am a post-doctoral researcher at Ghent University, affiliated with the Department of Applied Mathematics, Computer Science and Statistics (TWIST) as well as the Saeys Lab at the VIB Inflammation Research Center. I am also a teaching assistant for the Artificial Intelligence course offered by Ghent University at the Faculty of Sciences, as well as the lecturer for the Mathematics course in Biomedical Sciences.
My main focus of research is the study of adversarial examples. Broadly speaking, adversarial examples are input samples deliberately crafted by a malicious adversary in order to obtain certain specific predictions from a targeted machine learning model. The intent here is usually to cause some form of harm, such as bypassing automated content filters, malware protections or biometric security systems. In my work, I try to devise countermeasures against this form of exploitation.
Aside from research into adversarial examples, I am also interested in issues of fairness in machine learning. In developing and deploying machine learning systems, researchers and practitioners alike are often ignorant of (or deliberately ignore) the disparate impact of their systems on women and minority groups. Some of these tools, such as the recommender systems used by social media, also facilitate the spread of hate and political extremism across the globe. We cannot afford to remain blind to these problems; the field of machine learning must take its social and environmental impact seriously.
If you are a student or post-doc interested in working on robustness or interpretability of machine learning models, especially in the biomedical domain, check out our job postings. Spontaneous applications are also welcome; feel free to reach out to me with a cv and motivation letter.
latest posts
Aug 19, 2024 | A reverse Cantelli inequality |
---|---|
Jul 31, 2023 | Topological properties of adversarial examples |
Jun 26, 2023 | Fun with differentials |